R E S E A R C H
Research Interests
- Artificial Intelligence
- Machine Learning
- Medical applications of AI
- Computational Finance
Current Projects
The current focus in my lab is the application of Artificial Intelligence technology to Medicine. There are 3 active projects:
- Using AI to detect Heart Failure and other conditions based on videos of the so-called sniff test in Medicine. This is an ongoing collaboration with UCSF based on the largest annotated data set of its kind.
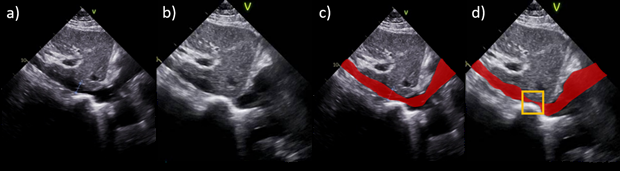
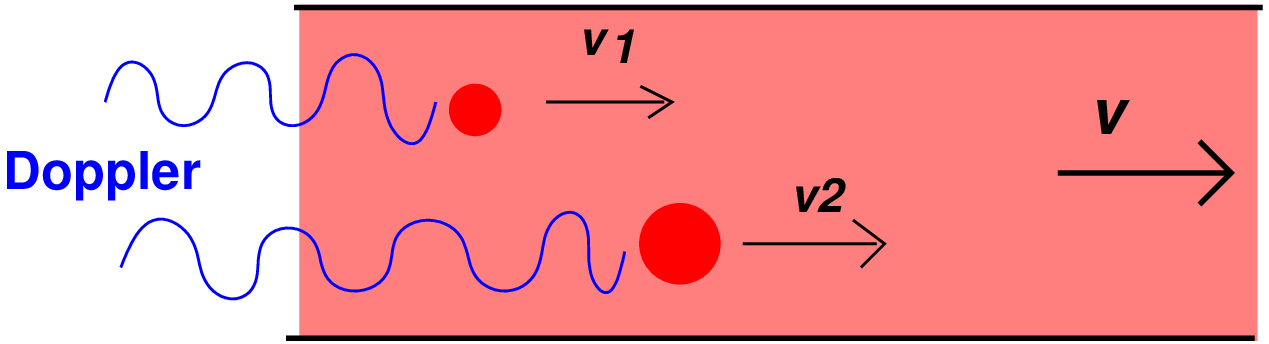
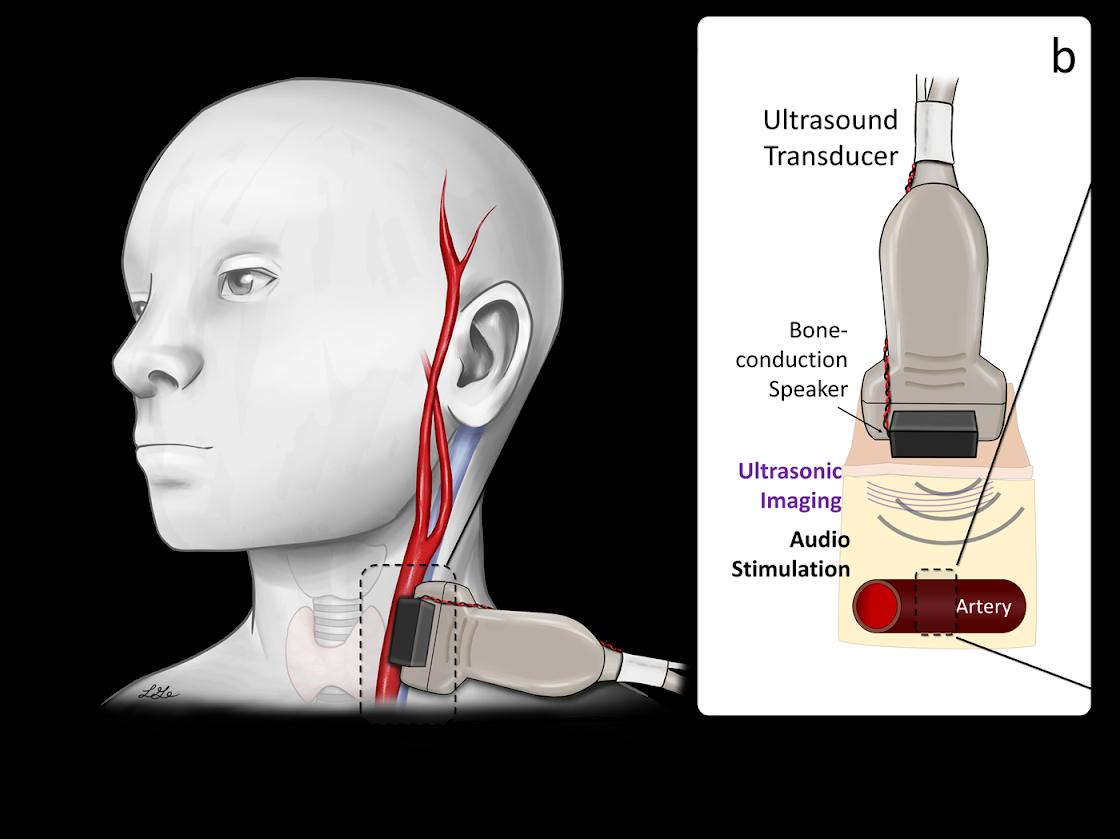
In all of these projects, AI/ML is an essential technology to deal with the realities of the human body that do not fit idealistic assumptions or lend themselves to conclusive mathematical analysis.
Another active project is the extension of the Learning from Hints method that was successful in financial applications to other applications based on recent revolutionary developments in AI. New generative techniques and advanced computational hardware show promise in making hints much more effective in improving the performance of neural networks in a wide range of applications.
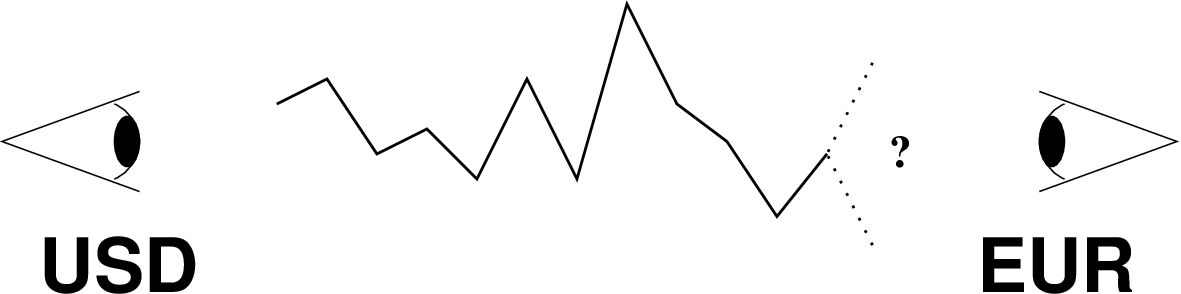
Previous Highlights
Some of the highlights of our past research in chronological order:
- Foundational work in AI based on early models of neural networks.
- The Learning From Hints paradigm.
- Neural Networks in the Capital Markets and Computational Finance.
- Theoretical analysis of learning based on the bin model.
- Learning from very noisy data.
- Application of AI to e-commerce and recommender systems.
- Transforming the training data optimally based on the distribution of the test inputs.
- Using AI to predict the daily spread of COVID-19 in every US county.